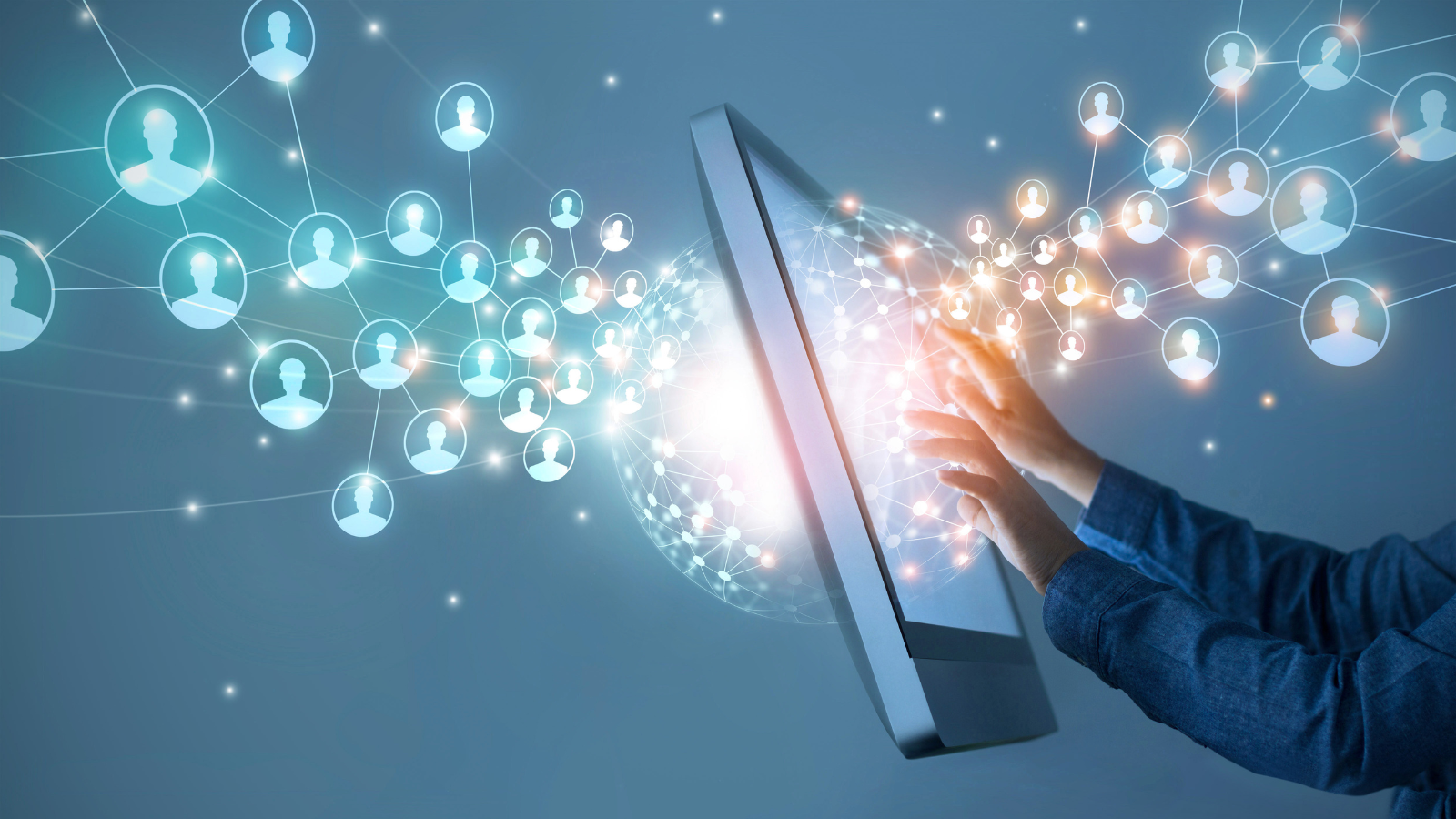
Red Eléctrica's transmission grid planning team is constantly interacting with a wide range of stakeholders such as public institutions, associations or platforms in the development of its activities. Managing conversations with different agents is a complex task because they are represented by different interlocutors. However, the positioning of these groups is of great importance for the company, so in order to communicate with them it is necessary to obtain a profile of each group.
For this purpose, a proof of concept was done with the Madrid-based startup Argilla, a company specialized in processing and organizing unstructured data using artificial intelligence and language processing solutions to help large companies analyze the huge flow of information they daily generate, automating text analysis and transforming massive data into accessible and easy-to-understand information.
In this project, which was born thanks to Elewit's venture client program, we worked on the automatic creation of stakeholder profiles of Red Eléctrica's transmission planning team from the analysis of communications in text format, taking advantage of Argilla's tool, Biome. The main objective was to obtain a clear idea of how the company connects with each stakeholder group, to ensure that communication is as effective and personalized as possible. To achieve this goal, a natural language processing (NLP) analysis of the content of various text communications was performed. The main drivers of the project were as follows:
- Acknowledging what stakeholders perceive and observe about Red Eléctrica.
- Achieving a global vision from the different stakeholders.
- Obtaining objective data which would enable a customed and dedicated relationship with each group.
For model training, a dataset was built so that the models could learn to classify and detect patterns that would identify both the perception of the stakeholders and the thematic categories themselves. In this sense, several versions of the model were generated during the project, trained in each interaction with a larger amount of data.