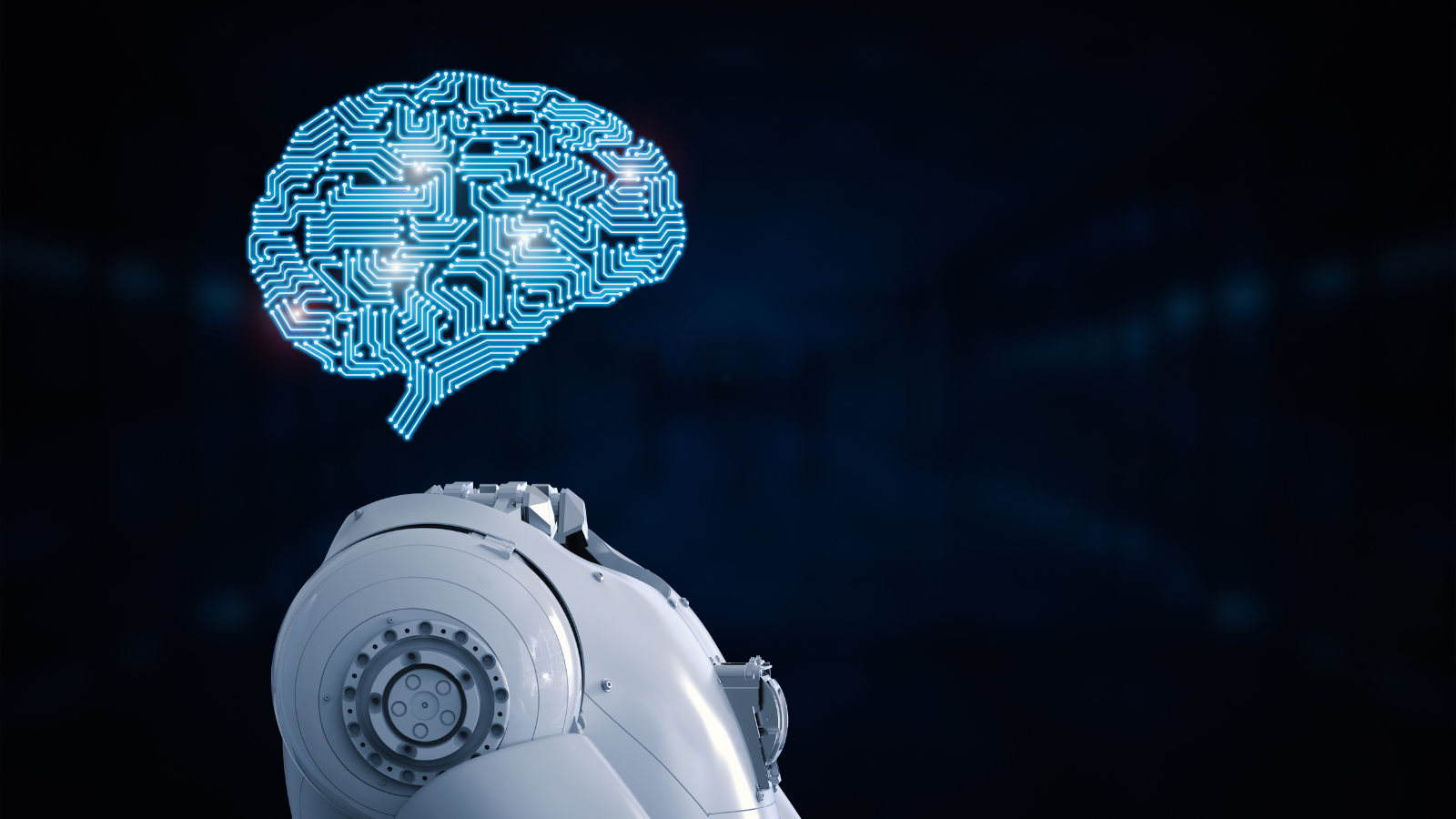
The management of critical infrastructures, such as the electrical grid, is facing increasingly complex challenges, and artificial intelligence (AI) could be the key to transforming the industry's efficiency and competitiveness. In this sense, AI has emerged in recent years as a disruptive technology with the potential to revolutionise how we manage these infrastructures. However, its implementation raises a series of challenges that must be addressed through the strict and meticulous application of principles, standards, and methodologies to ensure result precision and reliability.
Complexity and diversity of electricity transmission grids
The increasing complexity of the electrical grid presents challenges in terms of system modelling, planning, simulation, and operation. Some key points to consider when implementing the use of AI include:
- The growing interconnection of physical and digital systems in electrical grids hinders the creation of precise and comprehensive models.
- The integration of renewable energy sources, such as solar and wind power, introduces significant variability in energy generation. This in turn complicates grid prediction and operation.
- Ensuring access to and availability of all required data across multiple systems is complex. Additionally, data formats and quality levels vary, making their integration and analysis challenging.
Cybersecurity
It is essential to recognise that the increasing reliance on interconnected digital systems exposes critical infrastructures to a high risk of cyberattacks. Moreover, attackers can use AI to develop more sophisticated and harder-to-detect attacks. The main types of cyberattacks include:
- Targeted attacks: attackers can use AI to develop highly personalised and hard-to-detect attacks, exploiting specific vulnerabilities in systems.
- Data poisoning: attackers can introduce false or manipulated data into data capture systems, leading to incorrect AI model results and harmful decision-making.
- Adversarial attacks: these attacks exploit vulnerabilities inherent to machine learning models. AI models are trained on large amounts of data and can be deceived by introducing slightly altered data, which leads the model to make incorrect predictions.
Resilience
Resilience in critical infrastructures, such as the electrical grid, refers to their ability to withstand, recover from, and adapt to external disruptions, whether natural or artificial. It is a system's ability to maintain its essential functions despite having sustained damage or stress. Some of the disruptions that AI must allow for include:
- Extreme events: natural phenomena that can cause significant damage to electrical infrastructures. In such situations, AI must be capable of adapting to these conditions.
- Cascading failures: a failure in one component of the grid can trigger a cascade of failures in other components, leading to a widespread service disruption. AI must be able to identify and mitigate these cascades.
- Disaster recovery: AI can help speed up grid recovery after a disaster, but it can also be affected by the same event.
Interpretability and explainability
In the field of artificial intelligence, interpretability refers to the ability to understand and explain how an AI model reaches a particular decision. This is essential as more complex AI models can be difficult to interpret. In this sense, interpretability becomes a crucial element in promoting AI implementation because:
- It fosters trust by allowing us to understand how a model reaches its results.
- It facilitates model debugging. In other words, if a model fails, interpretability helps identify and correct errors.
- It complies with current regulations requiring AI systems to be transparent and explainable, especially when it comes to decisions affecting critical systems.
Here, interpretability not only improves the reliability of AI models but also facilitates their development, responsible use, and compliance with regulations.
Scalability
Scalability is another key concept in the world of artificial intelligence, especially when discussing its application to large and complex systems like critical infrastructures. Specifically, scalability refers to the ability of an AI system to handle increased data volume, user count, or task complexity without a significant decline in performance. It is an essential feature for any artificial intelligence system because:
- AI systems are trained on massive datasets, making scalability crucial to process this data efficiently and extract valuable information.
- Applications must process large amounts of data in real-time, and scalability ensures the system can handle this workload.
- AI systems are not static; they evolve over time. Scalability allows for the integration of new data, models, and functionalities without the need to redesign the entire system.
On the other hand, scalability often presents a challenge, as it requires substantial computing power and storage capacity. Moreover, some AI algorithms are particularly difficult to scale. Finally, it is important to consider that efficient communication between different nodes is essential for scalability in distributed systems.
Benefits and risks of human-machine collaboration
It's no secret that AI systems provide significant support in managing critical infrastructures by enhancing human capabilities through the automation of repetitive tasks and the processing of large amounts of data. As a result, humans can make more informed and precise decisions. Additionally, AI is creating new forms of interaction between humans and machines, such as virtual assistants and chatbots.
Human-machine collaboration offers numerous benefits, such as improved outcomes, efficiency, and accuracy. However, we must also consider the challenges it presents, including data biases and ethical implications. It is crucial to ensure that AI is used responsibly and transparently, as managing large amounts of personal and sensitive data presents significant challenges in terms of privacy and ethics.